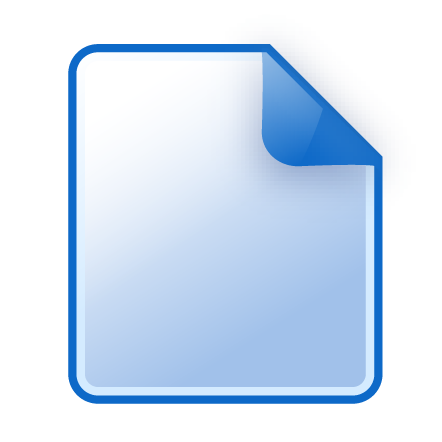
Actions
Export to: EndNote | Zotero | Mendeley
Collections
This file is not currently in any collections.
Global Scale Attention-based Deep Learning for river landscape classification [dataset] Open Access
This dataset is composed of a series of images and manually labelled masks. The goal is to train and test semantic classification models for riverine landscapes. In the masks, class 1 is rivers, class 2 is lakes and class 3 is sediment bars. All files are in geotif format and will open directly in any geospatial software package (QGIS, ArcGIS, etc..).
Descriptions
- Resource type
- Dataset
- Contributors
- Creator:
Carbonneau, Patrice
1
1 Durham University, UK
- Funder
- Research methods
-
Semantic Image classification with Deep Learning
- Other description
-
The main zip file contains 3 archives: SeenTrainingTiles.xz which incudes 610 pairs of images and masks and is intended to provide training and validation data. SeenTestingTiles.xz has 67 pairs iof images and masks drawn from the same geographical locations as the above training tiles. It is intended as a preliminary test dataset. UnseenTestTiles.xz has an additional 100 pairs of images and masks and is intented as an independent testing set never seen in the training stages of the model. Each tile covers 0.15 x 0.15 degrees in area. The imagery is taken from the Sentinel-2 satellite and has 3 channels of NIR (band 8), Red (band 4) and green (band 3)
- Keyword
- Attention mechanism
Unet
Riverine landscapes
- Subject
-
Geography
- Location
- Language
- Cited in
- Identifier
- ark:/32150/r36682x393c
doi:10.15128/r36682x393c
- Rights
- Creative Commons Attribution 4.0 International (CC BY)
- Publisher
-
Durham University
- Date Created
File Details
- Depositor
- P. Carbonneau
- Date Uploaded
- 17 September 2024, 13:09:58
- Date Modified
- 17 September 2024, 14:09:08
- Audit Status
- Audits have not yet been run on this file.
- Characterization
- not yet characterized
User Activity | Date |
---|---|
User N. Syrotiuk has updated Global Scale Attention-based Deep Learning for river landscape classification [dataset] | 8 months ago |