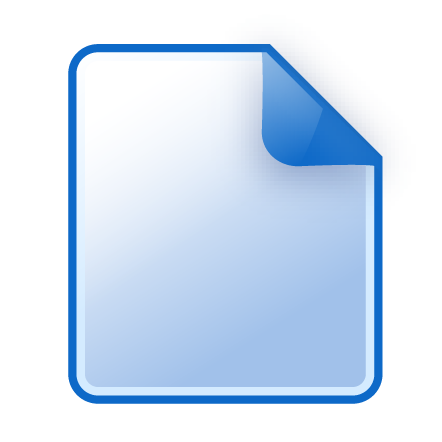
Actions
Export to: EndNote | Zotero | Mendeley
Collections
This file is not currently in any collections.
Cross-Domain Structure Preserving Projection for Heterogeneous Domain Adaptation [dataset] Open Access
Heterogeneous Domain Adaptation (HDA) addresses the transfer learning problems where data from the source and target domains are of different modalities (e.g., texts and images) or feature dimensions (e.g., features extracted with different methods). It is useful for multi-modal data analysis. Traditional domain adaptation algorithms assume that the representations of source and target samples reside in the same feature space, hence are likely to fail in solving the heterogeneous domain adaptation problem. Contemporary state-of-the-art HDA approaches are usually composed of complex optimization objectives for favourable performance and are therefore computationally expensive and less generalizable. To address these issues, we propose a novel Cross-Domain Structure Preserving Projection (CDSPP) algorithm for HDA. As an extension of the classic LPP to heterogeneous domains, CDSPP aims to learn domain-specific projection to map sample features from source and target domains into a common subspace such that the class consistency is preserved and data distributions are sufficiently aligned. CDSPP is simple and has deterministic solutions by solving a generalized eigenvalue problem. It is naturally suitable for supervised HDA but has also been extended for semi-supervised HDA where the unlabelled target domain samples are available. Extensive experiments have been conducted on commonly used benchmark datasets (i.e. Office-Caltech, Multilingual Reuters Collection, NUS-WIDE-ImageNet) for HDA as well as the Office-Home dataset firstly introduced for HDA by ourselves due to its significantly larger number of classes than the existing ones (65 vs 10, 6 and 8). The experimental results of both supervised and semi-supervised HDA demonstrate the superior performance of our proposed method against contemporary state-of-the-art methods. Supporting dataset for: Cross-Domain Structure Preserving Projection for Heterogeneous Domain Adaptation (Q. Wang, T.P. Breckon), In Pattern Recognition, Elsevier, Volume 123, 2022. DOI: https://doi.org/10.1016/j.patcog.2021.108362 DRO: https://dro.dur.ac.uk/34127/
Descriptions
- Resource type
- Dataset
- Contributors
- Data curator:
Wang, Qian
1
Creator: Breckon, Toby 1
Editor: Breckon, Toby 1
1 Durham University, UK
- Funder
-
Durham University, UK
- Research methods
-
empirical machine learning
- Other description
-
Supporting open source software: https://github.com/hellowangqian/cdspp-hda
- Keyword
- heterogeneous domain adaptation
cross-domain projection
image classification
text classification
machine learning
- Subject
-
Neural networks (Computer science)
Artificial intelligence
- Location
-
Durham, England, United Kingdom
- Language
- English
- Cited in
- doi:10.1016/j.patcog.2021.108362
- Identifier
- ark:/32150/r2jw827b67n
doi:10.15128/r2jw827b67n
- Rights
- Creative Commons Attribution 4.0 International (CC BY)
- Publisher
-
Durham University
- Date Created
-
February 2022
File Details
- Depositor
- T. Breckon
- Date Uploaded
- 30 May 2022, 22:05:04
- Date Modified
- 31 May 2022, 14:05:10
- Audit Status
- Audits have not yet been run on this file.
- Characterization
-
File format: zip (ZIP Format)
Mime type: application/zip
File size: 1458559618
Last modified: 2022:05:30 23:50:48+01:00
Filename: UDA-features.zip
Original checksum: e90e26121a4df03bca56af61c9300b95
User Activity | Date |
---|---|
User T. Breckon has updated Cross-Domain Structure Preserving Projection for Heterogeneous Domain Adaptation - Supporting Dataset | almost 3 years ago |
User T. Breckon has updated Cross-Domain Structure Preserving Projection for Heterogeneous Domain Adaptation - Supporting Dataset | almost 3 years ago |
User T. Breckon has updated Cross-Domain Structure Preserving Projection for Heterogeneous Domain Adaptation - Supporting Dataset | almost 3 years ago |
User T. Breckon has deposited UDA-features.zip | almost 3 years ago |