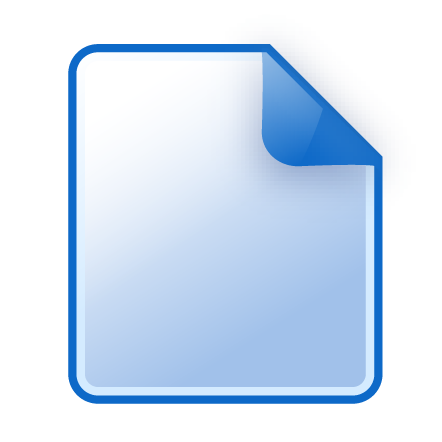
Actions
Export to: EndNote | Zotero | Mendeley
Collections
This file is not currently in any collections.
Coupling Molecular Dynamics and Deep Learning to Mine Protein Conformational Space [data and software] Open Access
Descriptions
- Resource type
- Software
- Contributors
- Creator:
Degiacomi, Matteo
1
Contact person: Degiacomi, Matteo 1
Data collector: Degiacomi, Matteo 1
Data curator: Degiacomi, Matteo 1
Editor: Degiacomi, Matteo 1
1 Durham University, UK
- Funder
-
Engineering and Physical Sciences Research Council
- Research methods
- Other description
-
This work features generative neural networks (autoencoders) trained on protein structures produced by molecular simulations. Autoencoders are used to obtain new, plausible conformations complementing and extending pre-existing ones, usable in a protein-protein docking scenario.
- Keyword
- molecular modelling
deep learning
molecular dynamics
proteins
- Subject
-
Molecular dynamics
Proteins
Neural networks (Computer science)
- Location
- Language
- English
- Cited in
- Identifier
- ark:/32150/r26w924b81m
doi:10.15128/r26w924b81m
- Rights
- GNU General Public Licence 3 (GPL-3.0)
- Publisher
-
Durham University
- Date Created
File Details
- Depositor
- M.T. Degiacomi
- Date Uploaded
- 11 March 2019, 17:03:57
- Date Modified
- 23 March 2019, 11:03:58
- Audit Status
- Audits have not yet been run on this file.
- Characterization
-
File format: x-gzip (GZIP Format, GZIP)
Mime type: application/x-gzip
File size: 1236676114
Last modified: 2019:03:11 17:40:59+00:00
Filename: Degiacomi_Structure_2019.tar.gz
Original checksum: 21fa4248c866f632c9d5a49828e20cd1
User Activity | Date |
---|---|
User M.T. Degiacomi has updated Coupling Molecular Dynamics and Deep Learning to Mine Protein Conformational Space [data and software] | over 6 years ago |
User N. Syrotiuk has updated Coupling Molecular Dynamics and Deep Learning to Mine Protein Conformational Space [data and software] | over 6 years ago |
User M.T. Degiacomi has updated Coupling Molecular Dynamics and Deep Learning to Mine Protein Conformational Space [data and software] | over 6 years ago |
User M.T. Degiacomi has updated Coupling Molecular Dynamics and Deep Learning to Mine Protein Conformational Space [data and software] | over 6 years ago |
User M.T. Degiacomi has deposited Degiacomi_Structure_2019.tar.gz | over 6 years ago |