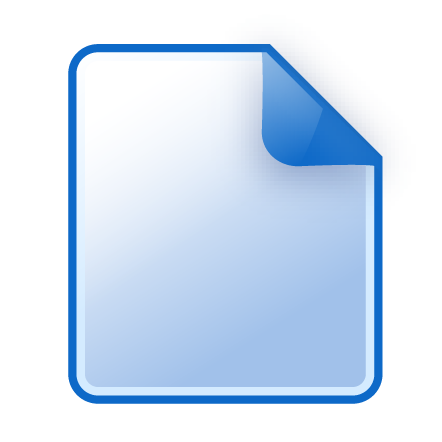
Actions
Export to: EndNote | Zotero | Mendeley
Collections
This file is in the following collections:
On The Impact Of Varying Region Proposal Strategies For Raindrop Detection And Classification Using Convolutional Neural Networks - Supporting Materials |
Pretrained Neural Network Models for Guo 2018 study - TensorFlow format Open Access
The presence of raindrop induced image distortion has a significant negative impact on the performance of a wide range of all-weather visual sensing applications including within the increasingly important contexts of visual surveillance and vehicle autonomy. A key part of this problem is robust raindrop detection such that the potential for performance degradation in effected image regions can be identified. Here we address the problem of raindrop detection in colour video imagery by considering three varying region proposal approaches with secondary classification via a number of novel convolutional neural network architecture variants. This is verified over an extensive dataset with in-frame raindrop annotation to achieve maximal 0.95 detection accuracy with minimal false positives compared to prior work. Our approach is evaluated under a range of environmental conditions typical of all-weather automotive visual sensing applications. | Cited in: n The Impact Of Varying Region Proposal Strategies For Raindrop Detection And Classification Using Convolutional Neural Networks (T. Guo, S. Akcay, P. Adey, T.P. Breckon), In Proc. International Conference on Image Processing, IEEE, pp. 3413-3417, 2018. | This collection contains supporting materials in the form of the pre-trained network models and image data used in the study.
Descriptions
- Resource type
- Dataset
- Contributors
- Creator:
Guo, Tiancheng
1
Data collector: Guo, Tiancheng 1
Data curator: Guo, Tiancheng 1
Contact person: Breckon, Toby 1
Data curator: Breckon, Toby 1
Editor: Breckon, Toby 1
1 Durham University, UK
- Funder
- Research methods
- Other description
- Keyword
- Computer vision
Raindrop detection
Rain detection
Rain removal
Rain noise removal
Rain interference
Scene context
Raindrop saliency
Rain classification
Convolutional neural network (CNN)
Deep learning
- Subject
-
Computer vision
Rain and rainfall
Neural networks (Computer science)
Computer Science
Engineering
- Location
- Language
- Cited in
- Identifier
- ark:/32150/r23j333226h
doi:10.15128/r23j333226h
- Rights
- MIT Licence (MIT)
- Publisher
-
Durham University
- Date Created
File Details
- Depositor
- T. Breckon
- Date Uploaded
- 25 November 2018, 16:11:54
- Date Modified
- 29 November 2018, 12:11:57
- Audit Status
- Audits have not yet been run on this file.
- Related Files
- guo-raindrop-detection-dataset-2018.zip
- Characterization
-
File format: zip (ZIP Format)
Mime type: application/zip
File size: 300038803
Last modified: 2018:11:27 23:14:31+00:00
Filename: guo-raindrop-detection-pretrained-model-2018.zip
Original checksum: ea691f2538f1d2e0de57a108b4285173
User Activity | Date |
---|---|
User N. Syrotiuk has updated Pretrained Neural Network Models for Guo 2018 study - TensorFlow format | over 5 years ago |
User T. Breckon has added a new version of Pretrained Neural Network Models for Guo 2018 study - TensorFlow format | over 5 years ago |