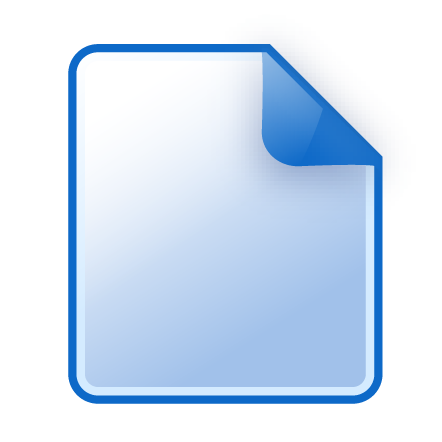
Actions
Export to: EndNote | Zotero | Mendeley
Collections
This file is not currently in any collections.
Fuzzy Classification of Sentinel 2 data with UAV-derived label data [dataset] Open Access
An estimated 76% of the world’s stream area is occupied by channels with widths above 30-45 meters. Sentinel 2 image data with resolutions of 10m could therefore supply information about the composition of riparian corridors at national and global scales. Fuzzy classification models that infer sub-pixel composition could further be used to compensate for small channel widths. One barrier to such work is the acquisition of suitable training data useable in machine learning models that can predict land-cover type information from image radiance values. In this short contribution, we present a method which combines UAV and Sentinel 2 imagery in order to develop a fuzzy classification approach capable of large-scale investigations. Our approach rests on the use of hyperspatial UAV data in order to train fully fuzzy classification models for Sentinel 2 data where all bands are super-resolved to a spatial resolution of 10 meters. We test random forest models, dense neural networks and convolutional neural networks. Our convolutional neural network models can identify pixels in the river corridor purely occupied by water, vegetation or sediment to an accuracy of 95.5%. The model can then predict the composition of the remaining mixed-pixels with a mean error of 0% and an RMS error of 28%. We also find that the use of low-altitude UAV data improves classification performance with respect to a purely desk-based approach which relies on the user to interpret data on-screen without the benefit of field data. We therefore argue that the novel use of UAVs as field validation tools for freely available satellite data can bridge the scale gap between local and regional fluvial studies. This should allow for wider scale investigations of key processes such as basin scale sediment transport, vegetation dynamics or other processes currently in a state of flux due to anthropogenic impacts.
Descriptions
- Resource type
- Dataset
Image
- Contributors
- Data curator:
Carbonneau, Patrice
1
Data collector: Belletti, Barbara 2
Data collector: Micotti, Marco 2
Mariani, Stefano 3
Castelletti, Andrea 2
Bizzi, Simone 4
1 Durham University, UK
2 Politecnico Milano
3 ISPRA
4 Universita Padova
- Funder
-
Istituto Superiore per la Protezione e la Ricerca Ambienta, Rome,Italy
Geography Department, Durham University, Durham, UK
- Research methods
-
Machine Learning
- Other description
- Keyword
- Machine Learning
Fuzzy Classification
Sentinel 2
UAV
- Subject
-
Fuzzy Image Classification
- Location
- Language
- Cited in
- Identifier
- ark:/32150/r1v692t6239
- Rights
- MIT Licence (MIT)
- Publisher
-
Durham University
- Date Created
File Details
- Depositor
- P. Carbonneau
- Date Uploaded
- 5 February 2020, 12:02:25
- Date Modified
- 5 February 2020, 17:02:12
- Audit Status
- Audits have not yet been run on this file.
- Characterization
-
File format: zip (ZIP Format)
Mime type: application/zip
File size: 28739600
Last modified: 2020:04:29 11:25:23+01:00
Filename: UAV2SEN_DATA.zip
Original checksum: 1b47206c0cc534470549dee844825cb6
User Activity | Date |
---|---|
User N. Syrotiuk has added a new version of Fuzzy Classification of Sentinel 2 data with UAV-derived label data [dataset] | almost 5 years ago |
User P. Carbonneau has updated Fuzzy Classification of Sentinel 2 data with UAV-derived label data [dataset] | about 5 years ago |
User P. Carbonneau has updated Fuzzy Classification of Sentinel 2 data with UAV-derived label data [dataset] | about 5 years ago |
User P. Carbonneau has updated Fuzzy Classification of Sentinel 2 data with UAV-derived label data [dataset] | about 5 years ago |
User P. Carbonneau has deposited UAV2SEN_Data.zip | about 5 years ago |