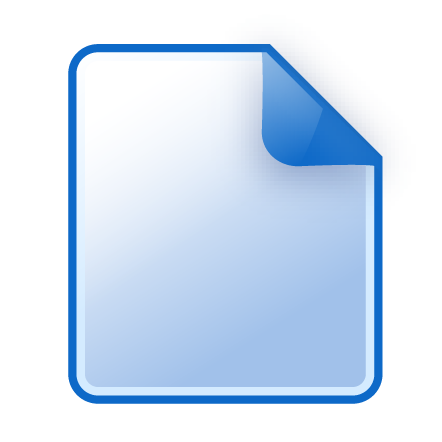
Actions
Export to: EndNote | Zotero | Mendeley
Collections
This file is in the following collections:
Efficient and Compact Convolutional Neural Network Architectures for Non-temporal Real-time Fire Detection - supporting materials |
Pretrained Neural Network Models for Thomson 2020 study - PyTorch format Open Access
Automatic visual fire detection is used to complement traditional fire detection sensor systems (smoke/heat). In this work, we investigate different Convolutional Neural Network (CNN) architectures and their variants for the nontemporal real-time bounds detection of fire pixel regions in video (or still) imagery. Two reduced complexity compact CNN architectures (NasNet-A-OnFire and ShuffleNetV2-OnFire) are proposed through experimental analysis to optimise the computational efficiency for this task. The results improve upon the current state-of-the-art solution for fire detection, achieving an accuracy of 95% for full-frame binary classification and 97% for superpixel localisation. We notably achieve a classification speed up by a factor of 2.3× for binary classification and 1.3× for superpixel localisation, with runtime of 40 fps and 18 fps respectively, outperforming prior work in the field presenting an efficient, robust and real-time solution for fire region detection. Subsequent implementation on low-powered devices (Nvidia Xavier-NX, achieving 49 fps for full-frame classification via ShuffleNetV2-OnFire) demonstrates our architectures are suitable for various real-world deployment applications. | Cited in: Efficient and Compact Convolutional Neural Network Architectures for Non-temporal Real-time Fire Detection (W. Thomson, N. Bhowmik, T.P. Breckon), In Proc. Int. Conf. on Machine Learning Applications, IEEE, 2020. | This file contains supporting materials in the form of the pre-trained network models used in the study.
Descriptions
- Resource type
- Other
- Contributors
- Creator:
Thomson, William
1
Contact person: Bhowmik, Neelanjan 1
Editor: Bhowmik, Neelanjan 1
Editor: Breckon, Toby P. 1
1 Durham University, UK
- Funder
-
Durham University
- Research methods
- Other description
- Keyword
- Convolutional Neural Network
fire detection
classification
- Subject
-
Engineering
Computer science
- Location
-
Durham, England, United Kingdom
- Language
- English
- Cited in
- Efficient and Compact Convolutional Neural Network Architectures for Non-temporal Real-time Fire Detection (W. Thomson, N. Bhowmik, T.P. Breckon), In Proc. Int. Conf. on Machine Learning Applications, IEEE, 2020
- Identifier
- ark:/32150/r1tb09j570z
doi:10.15128/r1tb09j570z
- Rights
- MIT Licence (MIT)
Creative Commons Attribution 4.0 International (CC BY)
- Publisher
-
Durham University
- Date Created
File Details
- Depositor
- N. Bhowmik
- Date Uploaded
- 18 January 2021, 19:01:56
- Date Modified
- 7 March 2021, 19:03:43
- Audit Status
- Audits have not yet been run on this file.
- Characterization
-
File format: zip (ZIP Format)
Mime type: application/zip
File size: 155995759
Last modified: 2021:03:07 19:17:00+00:00
Filename: thomson-2020-fire-detection-pretrained-models-pytorch--version2.zip
Original checksum: 5c7be76d7f8149be4057849c998ab214
User Activity | Date |
---|---|
User N. Bhowmik has updated Pretrained Neural Network Models for Thomson 2020 study - PyTorch format | over 3 years ago |
User N. Bhowmik has updated thomson-2020-fire-detection-pretrained-models-pytorch--version1.zip | over 3 years ago |
User N. Bhowmik has updated thomson-2020-fire-detection-pretrained-models-pytorch--version1.zip | over 3 years ago |
User N. Bhowmik has deposited thomson-2020-fire-detection-pretrained-models-pytorch--version1.zip | over 3 years ago |