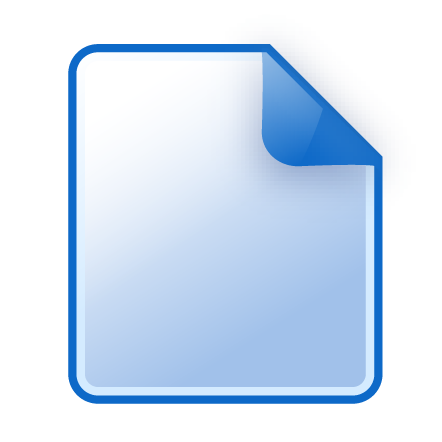
Actions
Export to: EndNote | Zotero | Mendeley
Collections
This file is not currently in any collections.
Extending Deep Neural Network Trail Navigation for Unmanned Aerial Vehicle Operation within the Forest Canopy - Evaluation [dataset] Open Access
Autonomous flight within a forest canopy represents a key challenge for generalised scene understanding on-board a future Unmanned Aerial Vehicle (UAV) platforms. Here we present an approach for automatic trail navigation within such an unstructured environment that successfully generalises across differing image resolutions - allowing UAV with a varying sensor payload capabilities to operate equally in such challenging environmental conditions. Specifically, this work presents an optimised deep neural network architecture, capable of state-of-the-art performance across varying resolution aerial UAV imagery, that improves forest trail detection for UAV guidance even when using significantly low resolution images that are representative of low-cost search and rescue capable UAV platforms. Used in the paper: Extending Deep Neural Network Trail Navigation for Unmanned Aerial Vehicle Operation within the Forest Canopy (B.G. Maciel-Pearson, P. Carbonneau, T.P. Breckon), In Proc. Towards Autonomous Robotic Systems Conference, Springer, 2018.
Descriptions
- Resource type
- Dataset
- Contributors
- Creator:
Maciel-Pearson, Bruna G.
1
Creator: Breckon, Toby P. 1
1 Durham University
- Funder
-
Engineering and Physical Sciences Research Council
- Research methods
- Other description
- Keyword
- Deep learning
Trail detection
Autonomous Unmanned Aerial Vehicle
Unstructured environment
Drone
Convolutional Neural Network
UAV
- Subject
-
Robotics
Deep Learning
Monocular Vision
Computer Science
Machine Learning
- Location
-
Durham, England, United Kingdom
- Language
- English
- Cited in
- Extending Deep Neural Network Trail Navigation for Unmanned Aerial Vehicle Operation within the Forest Canopy (B.G. Maciel-Pearson, P. Carbonneau, T.P. Breckon), In Proc. Towards Autonomous Robotic Systems Conference, Springer, pp. 1-11, 2018.
- Identifier
- ark:/32150/r1st74cq45z
doi:10.15128/r1st74cq45z
- Rights
- All rights reserved All rights reserved
- Publisher
-
Durham University
- Date Created
-
2018
File Details
- Depositor
- B.G. Maciel Pearson
- Date Uploaded
- 4 May 2018, 13:05:15
- Date Modified
- 4 May 2018, 13:05:23
- Audit Status
- Audits have not yet been run on this file.
- Characterization
-
File format: zip (ZIP Format)
Mime type: application/zip
File size: 1798571204
Last modified: 2018:05:04 13:24:35+01:00
Filename: UAVTrailNavigationDataset-Pearson-Breckon-2018-1211-0305-version3.zip
Original checksum: e4781cdfeb6cedbc9673de51c3c67094