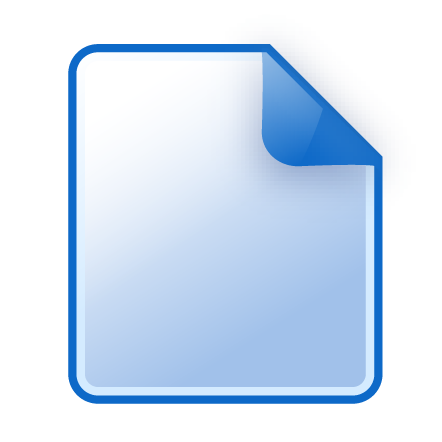
Actions
Export to: EndNote | Zotero | Mendeley
Collections
This file is not currently in any collections.
Generalized Zero-Shot Domain Adaptation via Coupled Conditional Variational Autoencoders [dataset] Open Access
Domain adaptation aims to exploit useful information from the source domain where annotated training data are easier to obtain to address a learning problem in the target domain where only limited or even no annotated data are available. In classification problems, domain adaptation has been studied under the assumption all classes are available in the target domain regardless of the annotations. However, a common situation where only a subset of classes in the target domain are available has not attracted much attention. In this paper, we formulate this particular domain adaptation problem within a generalized zero-shot learning framework by treating the labelled source-domain samples as semantic representations for zero-shot learning. For this novel problem, neither conventional domain adaptation approaches nor zero-shot learning algorithms directly apply. To solve this problem, we present a novel Coupled Conditional Variational Autoencoder (CCVAE) which can generate synthetic target-domain image features for unseen classes from real images in the source domain. Extensive experiments have been conducted on three domain adaptation datasets including a bespoke X-ray security checkpoint dataset to simulate a real-world application in aviation security. The results demonstrate the effectiveness of our proposed approach both against established benchmarks and in terms of real-world applicability.
Descriptions
- Resource type
- Dataset
- Contributors
- Creator:
Wang, Qian
1
Data collector: Wang, Qian 1
Data curator: Wang, Qian 1
Editor: Breckon, Toby 1
1 Durham University, UK
- Funder
-
Durham University, UK
- Research methods
-
Empirical machine learning
- Other description
-
Supporting open source software: https://github.com/hellowangqian/gzsda
Originally published 2023/05/22. New version published 2023/07/25 with corrections: (1) updated README.txt with full technical information on file formats and data set layout; (2) standardized file naming convention, independent of original file origin (scanner, internet etc); (3) error in README.txt file corrected to correctly attribute X-ray files across 2 different different source scanners.
- Keyword
- Generalized zero-shot learning
Conditional Variational Autoencoder
Domain adaptation
Generalized zero-shot domain adaptation
- Subject
-
Neural networks (Computer science)
Artificial intelligence
- Location
-
Durham, England, United Kingdom
- Language
- English
- Cited in
- doi:10.1016/j.neunet.2023.03.033
- Identifier
- ark:/32150/r1c534fn98x
doi:10.15128/r1c534fn98x
- Rights
- Creative Commons Attribution 4.0 International (CC BY)
- Publisher
-
Durham University
- Date Created
-
May 2023
File Details
- Depositor
- Q. Wang
- Date Uploaded
- 21 May 2023, 10:05:24
- Date Modified
- 25 July 2023, 10:07:37
- Audit Status
- Audits have not yet been run on this file.
- Characterization
-
File format: zip (ZIP Format)
Mime type: application/zip
File size: 1121055437
Last modified: 2023:07:25 10:02:18+01:00
Filename: ResearchData.zip
Original checksum: dd4f3da9f417d315982d7a59f1855af9
User Activity | Date |
---|---|
User N. Syrotiuk has added a new version of Generalized Zero-Shot Domain Adaptation via Coupled Conditional Variational Autoencoders [dataset] | almost 2 years ago |
User N. Syrotiuk has updated Generalized Zero-Shot Domain Adaptation via Coupled Conditional Variational Autoencoders [dataset] | almost 2 years ago |
User N. Syrotiuk has updated Generalized Zero-Shot Domain Adaptation via Coupled Conditional Variational Autoencoders [dataset] | almost 2 years ago |
User N. Syrotiuk has updated Generalized Zero-Shot Domain Adaptation via Coupled Conditional Variational Autoencoders [dataset] | almost 2 years ago |