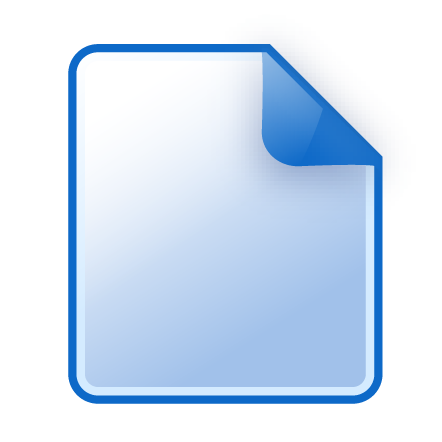
Actions
Export to: EndNote | Zotero | Mendeley
Collections
This file is not currently in any collections.
Re-ID-AR: Improved Person Re-identification in Video via Joint Weakly Supervised Action Recognition [dataset] Open Access
We uniquely consider the task of joint person re-identification (Re-ID) and action recognition in video as a multi-task problem. In addition to the broader potential of joint Re-ID and action recognition within the context of automated multi-camera surveillance, we show that the consideration of action recognition in addition to Re-ID results in a model that learns discriminative feature representations that both improve Re-ID performance and are capable of providing viable per-view (clip-wise) action recognition. Our approach uses a single 2D Convolutional Neural Network (CNN) architecture comprising a common ResNet50-IBN backbone CNN architecture, to extract frame-level features with subsequent temporal attention for clip-level feature extraction, followed by two subbranches:- the IDentification (sub-)Network (IDN) for person Re-ID and the ActionRecognition (sub-)Network for per-view action recognition. The IDN comprises a single fully connected layer while the ARN comprises multiple attention blocks on a one-to-one ratio with the number of actions to be recognised. This is subsequently trained as a jointRe-ID and action recognition task using a combination of two task-specific, multi-loss terms via weakly labelled actions obtained over two leading benchmark Re-ID datasets (MARS & LPW). Our consideration of Re-ID and action recognition as a multi-task problem results in a multi-branch 2D CNN architecture that outperforms prior work in the field (rank-1 (mAP) – MARS: 93.21%(87.23%), LPW: 79.60%) without any reliance 3D convolutions or multi-stream networks architectures as found in other contemporary work. Our work represents the first benchmark performance for such a joint Re-ID and action recognition video understanding task, hitherto unapproached in the literature, and is accompanied by a new public dataset of supplementary action labels for the seminal MARS and LPW Re-ID datasets.
Descriptions
- Resource type
- Dataset
- Contributors
- Creator:
Alsehaim, Aishah
1
Data collector: Alsehaim, Aishah 1
Breckon, Toby 1
1 Durham University, UK
- Funder
- Research methods
- Other description
- Keyword
- Re-identification
Action recognition
Convolutional Neural Networks
- Subject
-
Computer vision
Neural networks (Computer science)--Mathematical models
- Location
- Language
- Cited in
- https://dro.dur.ac.uk/34331/
- Identifier
- ark:/32150/r18c97kq420
doi:10.15128/r18c97kq420
- Rights
- Creative Commons Attribution 4.0 International (CC BY)
- Publisher
-
Durham University
- Date Created
File Details
- Depositor
- A.A. Alsehaim
- Date Uploaded
- 23 October 2021, 08:10:27
- Date Modified
- 11 November 2021, 12:11:38
- Audit Status
- Audits have not yet been run on this file.
- Characterization
-
File format: x-rar (RAR archive data, v8a, os: MS-DOS, Rar)
Mime type: application/x-rar
File size: 7159368
Filename: RE-ID-AR.rar
Original checksum: b88c253b97690d6edd8e5f34878139b3
User Activity | Date |
---|---|
User N. Syrotiuk has transferred Re-ID-AR: Improved Person Re-identification in Video via Joint Weakly Supervised Action Recognition [dataset] to user A.A. Alsehaim | over 3 years ago |